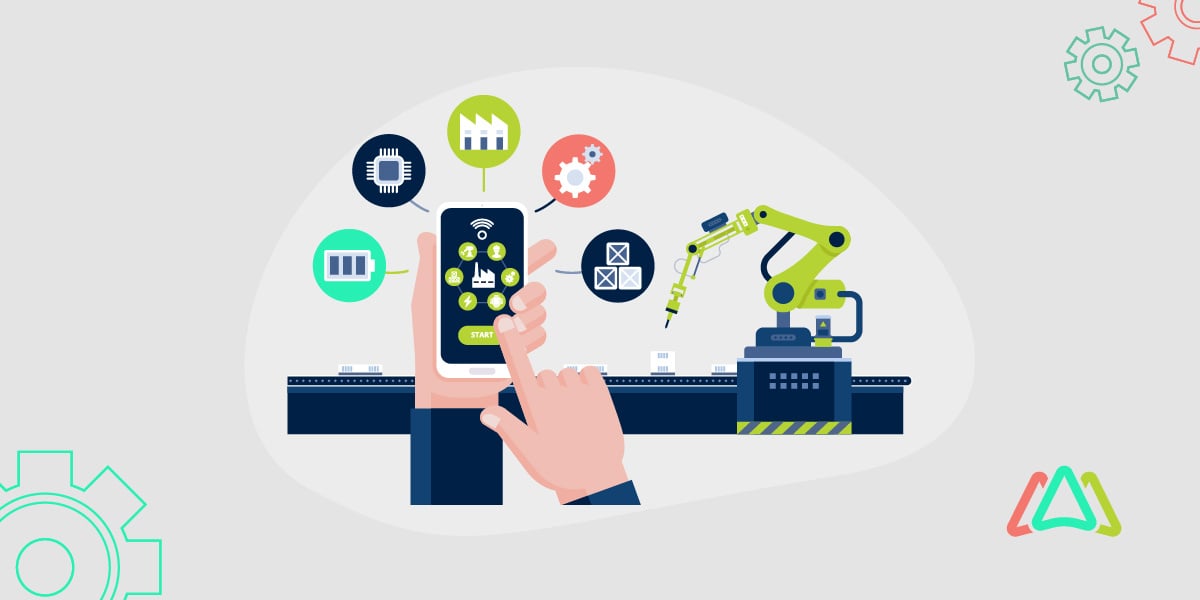
AI Enables Maintenance Management
Artificial intelligence (AI) talk has become commonplace.
Today, engaging in business-focused conversation without discussing AI is nearly impossible. This phenomenon extends beyond business, permeating everyday conversations as well. OpenAI's ChatGPT started this trend, leading to an explosion of AI technologies, including DeepSeek, Nvidia, Apple Intelligence, Google Gemini, and various other cloud services. The topic of AI is inescapable in business, with companies investing heavily in AI as the next productivity booster to sustain economic growth.
Compelling statistics underscore this trend. According to a PricewaterhouseCoopers (PwC) report, AI will have a $15.7 trillion economic impact by 2030. This figure surpasses the combined output of China and India. Of this total impact, $6.6 trillion is expected to result from increased productivity, accounting for nearly 40%, while the remaining 60% will come from consumption.
Although some market sectors and businesses are more advanced than others, AI remains in its early development stages overall. From a macroeconomic perspective, this presents opportunities for countries worldwide to leapfrog their more developed counterparts. On the other hand, developed economies are increasingly factoring AI gains into their GDP. The PwC report also highlights the potential impact of AI across various sectors, including healthcare, automotive, transport, logistics, and manufacturing.
The report identifies enhanced monitoring and auto-correction as high-potential use cases in manufacturing. Self-learning monitoring can make manufacturing processes more predictable and controllable, reducing costly delays, defects, or deviations from product specifications. The abundance of data throughout the manufacturing process enables intelligent monitoring. This sounds like a great productivity and efficiency enabler for the maintenance management function. However, barriers remain that need to be addressed.
This article explores AI's potential impact on maintenance management functions. It examines impact areas and how maintenance processes are improving. Additionally, it investigates work order management processes, which are central to maintenance management. This article captures how AI impacts maintenance management and what future trends maintenance professionals should watch out for.
Impact Areas - AI in Maintenance
AI has transformed various management functions, including maintenance management.
Through predictive analytics and automation, AI redefines business approaches to maintenance tasks, making them more efficient, cost-effective, and proactive. This transformative power of AI inspires optimism for the future of maintenance management by addressing skills issues and institutionalizing maintenance knowledge within companies.
Maintenance Efficiency
Predictive maintenance is the most prevalent feature of AI in maintenance management. Although other applications exist, predictive maintenance remains a strong use case for AI in this field. Predictive maintenance programs alone have delivered sustainable efficiency and cost savings. A guide from the US Department of Energy provides data on how predictive maintenance impacts efficiency and cost savings.
The advantages of predictive maintenance are many. A well-orchestrated predictive maintenance program can virtually eliminate costly equipment failures. It can schedule maintenance activities to minimize or eliminate overtime, minimize inventory, and order parts in advance to support upcoming planned maintenance. Optimizing equipment operation can reduce energy costs and increase plant reliability. Studies estimate that an effective predictive maintenance program can save 8% to 12% compared to using preventive maintenance. Depending on a facility's reliance on reactive maintenance, cost savings could exceed 30% to 40%.
Independent surveys indicate similar cost savings as a result of implementing an effective predictive maintenance program. Research published by Deloitte around predictive maintenance and innovative factory initiatives corroborates these findings.
- Return on investment: 10 times
- Reduction in maintenance costs: 25% to 30%
- Elimination of breakdowns: 70% to 75%
- Reduction in downtime: 35% to 45%
- Increase in production: 20% to 25%
However, starting a predictive maintenance program requires upfront capital expenditures and training of in-plant personnel to utilize predictive maintenance technologies. Additionally, a firm commitment from the organization and maintenance team backed by institutional support is necessary to make the program successful.
Technological Integration
The second factor is technological integration. It involves using AI with digital twins and Building Information Modeling (BIM) technologies. This integration enhances predictive analytics, allowing businesses to anticipate issues before they arise. The result is minimized downtime and extended asset lifespans. AI systems analyze vast data sets from various sources, transforming traditional reactive maintenance approaches into proactive strategies that lead to significant operational improvements.
Currently, in most organizations, dedicated teams perform analytics manually using spreadsheets and other tools, which limits their effectiveness. In contrast, AI can incorporate more variables and multiple data sets, enabling faster and more impactful analysis. Manual analysis often becomes outdated by the time it is executed.
Human Capital Challenges
Addressing human capital challenges and institutionalizing maintenance knowledge are both important. AI technologies are emerging at a pivotal time to solve pervasive problems in the maintenance sector from a human capital standpoint. The US Bureau of Labor Statistics projects that 3.1 million jobs in maintenance and repair occupations will be added between 2021 and 2031. A 2023 National Association of Manufacturers (NAM) report found that 28% of manufacturers expect over 25% of their workforce to retire within the next five years. These findings indicate a potential shortage of maintenance professionals to manage maintenance functions. AI’s automation capabilities will be essential for maintaining operational efficiency amid this projected shortage, providing reassurance for the future of maintenance management.
Artificial intelligence can help institutionalize maintenance knowledge as well. A recent Harris Poll survey revealed that 57% of working baby boomers have shared only half or less of the knowledge needed for their job responsibilities with successors. This indicates a significant knowledge transfer gap despite existing succession planning efforts. Senior technicians must impart decades of experience while performing their duties to train new employees effectively. AI is expected to address this challenge by ensuring faster knowledge transfer and creating an evolving body of maintenance knowledge that can be passed on during each transition. CMMS software is also a good starting point for addressing this challenge.
AI's integration with advanced technologies and its role in addressing workforce challenges will significantly impact maintenance management by enhancing predictive analytics and facilitating knowledge transfer within teams. This emphasis on AI's role in knowledge transfer instills confidence in the audience about the continuity of maintenance knowledge, even in the face of workforce changes.
The Context of AI in Maintenance
Before we examine how AI impacts maintenance processes, let's understand AI’s potential in maintenance management.
AI in maintenance primarily involves using machine learning (ML) algorithms, data analytics, and automation tools to streamline maintenance processes. Traditionally, maintenance has been reactive or scheduled at fixed intervals, often leading to unforeseen breakdowns and excessive downtime. AI shifts this paradigm toward predictive and prescriptive maintenance, allowing organizations to anticipate issues before they occur.
So, AI in maintenance has been chiefly concerned with predictive maintenance. However, it is progressing to newer areas, such as remote monitoring, automated maintenance scheduling, and CMMS using generative AI, including NLP. The following is a list of some AI components related to maintenance management.
- Predictive Maintenance
- Condition Monitoring
- Automated Scheduling
- Resource Allocation
- Generative AI
How is the Evolution of AI Impacting Maintenance Practices?
The rapid evolution of artificial intelligence (AI) is profoundly reshaping maintenance practices across diverse industries. From manufacturing to healthcare, AI technologies are enhancing operational efficiency, improving safety, and enabling proactive strategies. As organizations increasingly adopt AI-driven solutions, the impact on maintenance practices becomes clear. Here’s a detailed look at how AI evolution transforms maintenance practices.
Transition from Reactive to Proactive Maintenance
Predictive maintenance accelerates the transition from reactive to proactive maintenance. This section explains the central role of condition monitoring and predictive analytics.
Every business reliant on equipment and machinery, whether in manufacturing, logistics, energy, or facilities, faces the same dilemma: determining the appropriate time for maintenance. Conducting maintenance too early or too often wastes resources on unnecessary tasks, while performing it too late or not enough risks costly breakdowns, lost productivity, downtime, safety hazards, and compliance issues.
The challenge extends beyond merely keeping machines operational; it involves optimizing the timing and methods of maintenance. Traditionally, maintenance strategies have been categorized into reactive and preventive. Reactive maintenance involves fixing equipment only when it breaks down. Preventive maintenance follows a fixed schedule for servicing equipment, often resulting in replacing parts or performing maintenance that may not be necessary. For example, car maintenance typically follows a fixed schedule, which sometimes feels unnecessary as the car could have operated for additional miles without service. What if the vehicle could tell us when maintenance services are needed? In comes, condition monitoring.
Condition-Based Monitoring
Condition-based monitoring is fundamental to this process. Sensors installed on equipment continuously track key parameters such as temperature, vibration, motor speed, and electrical current, providing real-time insights into machine health. This data is fed into AI models that identify patterns and detect anomalies. If a machine exhibits unusual behavior, the system alerts maintenance and reliability staff to act before a breakdown occurs.
AI continuously analyzes real-time data from sensors installed on equipment through condition-based monitoring. Maintenance is performed when specific indicators suggest a fault rather than on a fixed schedule. This approach, backed by analytics, optimizes maintenance resources and enhances equipment reliability.
Predictive Maintenance Analytics
Predictive maintenance offers a solution by enabling equipment to indicate when attention is needed. Predictive maintenance employs real-time sensor data, AI-driven analytics, and machine learning models to predict equipment failure. This approach surpasses traditional methods by providing a data-driven, cost-effective, and intelligent way to manage industrial assets.
Analytics are at the core of predictive maintenance. The evolution of AI introduces predictive analytics that leverage historical data and machine learning algorithms to forecast potential issues. This proactive approach allows maintenance teams to schedule interventions based on predicted needs, reducing the risk of failure. Predictive maintenance enables scheduling based on anticipated needs, eliminating unnecessary maintenance while reducing unexpected downtimes and associated costs.
Condition-based monitoring and predictive analytics drive predictive maintenance, facilitating the transition from reactive to proactive maintenance.
Enhanced Decision-Making Capabilities
Maintenance decision-making has rarely been scientific. Many large enterprises and excellence-focused businesses only perform maintenance during breakdowns. Even with the development of predictive maintenance schedules, maintenance was more routine than decision-oriented. AI has the potential to greatly enhance maintenance decision-making greatly.
Data-Driven Insights
AI's capability to process and analyze large volumes of data provides maintenance teams with critical insights into equipment performance and potential issues. By utilizing machine learning models, organizations can identify patterns and anomalies that may not be visible through traditional analysis. This data-driven decision-making facilitates more informed strategies regarding maintenance schedules and resource allocation.
AI can examine historical work order data to identify trends and patterns in maintenance activities. This analysis can reveal inefficiencies, recurring issues, or common delays, enabling organizations to refine their processes and improve future maintenance strategies. More research is needed to determine the required level of human involvement in decision-making.
Root Cause Analysis
When equipment fails, AI can assist in root cause analysis, helping teams understand what went wrong and why it happened. By analyzing data from past failures, AI can identify contributing factors and suggest preventive measures, ultimately leading to more potent maintenance strategies. Businesses running CMMS software are better placed to take the next step of leveraging AI for root cause analysis. A root cause analysis is unheard of in most setups, owing to limited knowledge and lack of scale.
Improved Resource Allocation
The power of AI's analytics opens up several improvement opportunities in the maintenance function. The benefits derived from AI analytics pervade the maintenance function.
Intelligent Scheduling
Poor scheduling is a known cause of waste in the maintenance function. If the maintenance technician is unavailable, the spare will not be available, and vice versa.
AI technologies can optimize the scheduling of maintenance tasks by considering multiple variables, such as technician availability, equipment criticality, and job complexity. AI can intelligently assign the right personnel to specific work orders by analyzing skills, availability, and past performance. This ensures that tasks are completed efficiently and effectively, leveraging the strengths of individual team members while reducing onboarding times for complex tasks.
Inventory Management Integration
AI can enhance inventory management by connecting work order systems with inventory levels. This integration ensures that the necessary parts and materials are available when scheduled maintenance tasks are due, avoiding delays caused by material shortages and reducing downtime. AI can link work order systems with inventory management, ensuring that the necessary parts or materials are available when needed. When a work order is generated, the system can automatically check inventory levels and reorder supplies as required, reducing downtime due to material shortages. ERP systems have delivered this over the years, but it has become a feature exclusive to enterprise customers.
Work Order Automation
Work order management is an integral part of maintenance management. Work orders serve as the primary documentation for maintenance tasks and activities. They provide detailed instructions for maintenance personnel on what repairs or tasks need to be completed, along with relevant information such as timelines, locations, procedures, and the materials required. Many maintenance teams still manage work orders manually using pen, paper, and spreadsheets. Many maintenance departments use a CMMS or a point solution to manage reactive and planned preventive maintenance, but for most, these solutions still require some level of manual intervention.
AI is significantly transforming how work orders are created, managed, and executed, leading to improved efficiency and better resource utilization. Generative AI finds increased relevance in automating work order management. Here are several ways AI can revolutionize work order management:
Automated Work Order Generation
AI can automate the creation of work orders based on real-time data from IoT sensors and predictive maintenance analytics. When an anomaly or failure is detected, the system can automatically generate a work order specifying the tasks required to deal with the issue. This reduces manual effort and ensures timely interventions.
Prioritization of Work Orders
Prioritization is almost always missing when it comes to conventional work order management. AI algorithms can analyze data to prioritize work orders based on factors such as the criticality of the equipment, safety implications, and historical failure patterns. By automatically ranking tasks, maintenance teams can focus on the most urgent and impactful issues, improving overall operational efficiency.
Enhanced Work Order Tracking
AI-driven systems can provide real-time tracking of work orders, allowing maintenance managers to monitor progress and completion status. This visibility helps in understanding workloads, identifying bottlenecks, and making informed decisions about resource allocation and scheduling.
Facilitating Mobile Access to Work Orders
Thanks to the increasing computing power of mobile devices, AI functions are able to scale to smartphones, tablets, and other mobile devices. AI-powered mobile apps can enhance work order management by allowing technicians to access work orders, update statuses, and submit feedback while in the field. This real-time communication (enabled by nudges, voice commands, and technician behavior) reduces delays in information transfer and allows for quicker adjustments to work plans as conditions change.
Improved Communication and Collaboration
AI tools can enhance communication between maintenance teams, management, and other stakeholders involved in the work order process. By providing a centralized platform for sharing updates and documentation, AI promotes collaboration and ensures that everyone is aligned on maintenance activities.
Continuous Improvement and Learning
AI is poised to generate maximum impact for maintenance in this area. Earlier in this article, the shortage of maintenance talent was highlighted as a significant challenge. Coupled with this is the problem of institutionalizing maintenance knowledge within the organization to facilitate handovers during succession planning. AI is built to address these challenges by building maintenance knowledge in organizations.
Adaptive Learning Systems
The evolution of AI includes the development of systems that continuously learn from new data inputs. This adaptability allows maintenance practices to evolve based on historical trends and emerging technologies, leading to ongoing improvements and refinements in maintenance strategies.
Benchmarking and Performance Evaluation
AI systems can benchmark maintenance performance against industry standards and internal metrics. This ongoing evaluation helps organizations identify areas for improvement and recommend steps to remedy weaknesses and vulnerabilities, driving continuous enhancement of maintenance practices.
Training and Simulation
AI-powered simulations and augmented reality (AR) applications can improve maintenance technicians' training. These tools provide hands-on experience in a controlled setting, allowing technicians to practice under realistic scenarios without risking safety or equipment damage.
The AI Maintenance Management Technology is Just Getting Started.
The overall AI technology market is just getting started. Investment dollars in AI are staggering.
According to research from JP Morgan Asset Management, the five big hyperscalers (Microsoft, Alphabet, Meta, Amazon, and Oracle) poured an estimated $197 billion into AI infrastructure in 2024, with spending set to rise further. To support and train AI models, companies are making substantial investments in data centers, GPUs, and cloud-based infrastructure. Microsoft alone is anticipated to allocate $80 billion toward data centers in 2025.
It is expected that AI maintenance management use cases will only follow these larger AI developments. From available data related to the predictive maintenance market, we can get a sense of the direction and the scale.
In 2024, the global predictive maintenance market reached USD 12.7 billion, according to IMARC Group, and USD 7.24 billion, as reported by Precedence Research. Looking ahead, IMARC Group projects the market will grow to USD 80.6 billion by 2033, with a CAGR of 22.8% from 2025 to 2033. Precedence Research estimates an increase to USD 79.7 billion by 2034, with a CAGR of 27.1% between 2024 and 2034. This growth is driven by the rising adoption of machine-to-machine (M2M) communication and the integration of remote monitoring systems for advanced inspections.
A 25% CAGR over ten years for predictive maintenance is a bold bet. However, if we consider other evolving use cases for AI in maintenance, maintenance management will soon make inroads into the AI conversation.
TABLE OF CONTENTS
Keep Reading
Ever find yourself checking into a luxury hotel and expecting a relaxing stay, only to find a ...
11 Apr 2025
Organizations are witnessing swift changes in the business environment and confronting a ...
8 Apr 2025
Last month, news outlets and the entire internet was abuzz with the return of NASA astronauts ...
3 Apr 2025
What comes first - CMMS or predictive maintenance? If your answer is either, it is correct. ...
28 Mar 2025
Imagine a world where machines predict, diagnose, and fix their issues before they fail. This ...
25 Mar 2025
A facility maintenance plan is at the core of a facility’s operations. This organized ...
21 Mar 2025
Think of managing your maintenance operations like managing a championship sports team. Just ...
21 Mar 2025
The maintenance sector is battling a severe talent shortage that threatens to undermine ...
7 Mar 2025
Manufacturing maintenance is the backbone of industrial efficiency, ensuring machines run ...
5 Mar 2025
No one likes playing a guessing game when equipment breaks down. Yet, maintenance teams often ...
4 Mar 2025
The size of the preventive maintenance software market is discussed in millions of dollars, ...
4 Mar 2025
The organizational structure and corporate hierarchy vary from company to company. Large ...
28 Feb 2025
Maintenance procedures are essential for ensuring the longevity and reliability of machinery ...
21 Feb 2025
Sustainability is no longer just a buzzword; it's a critical component of corporate social ...
20 Feb 2025
A Computerized Maintenance Management System (CMMS) relies on accurate, well-organized data ...
18 Feb 2025
In an era where technology drives operational efficiency, Computerized Maintenance Management ...
14 Feb 2025
A Computerized Maintenance Management System (CMMS) is a key component of modern maintenance ...
13 Feb 2025
Introduction Maintenance management is the foundation of maintenance operations in industries ...
11 Feb 2025
Introduction A Computerized Maintenance Management System (CMMS) is software designed to help ...
7 Feb 2025
Introduction Due to a growing awareness of the need to combat climate change, the green ...
6 Feb 2025